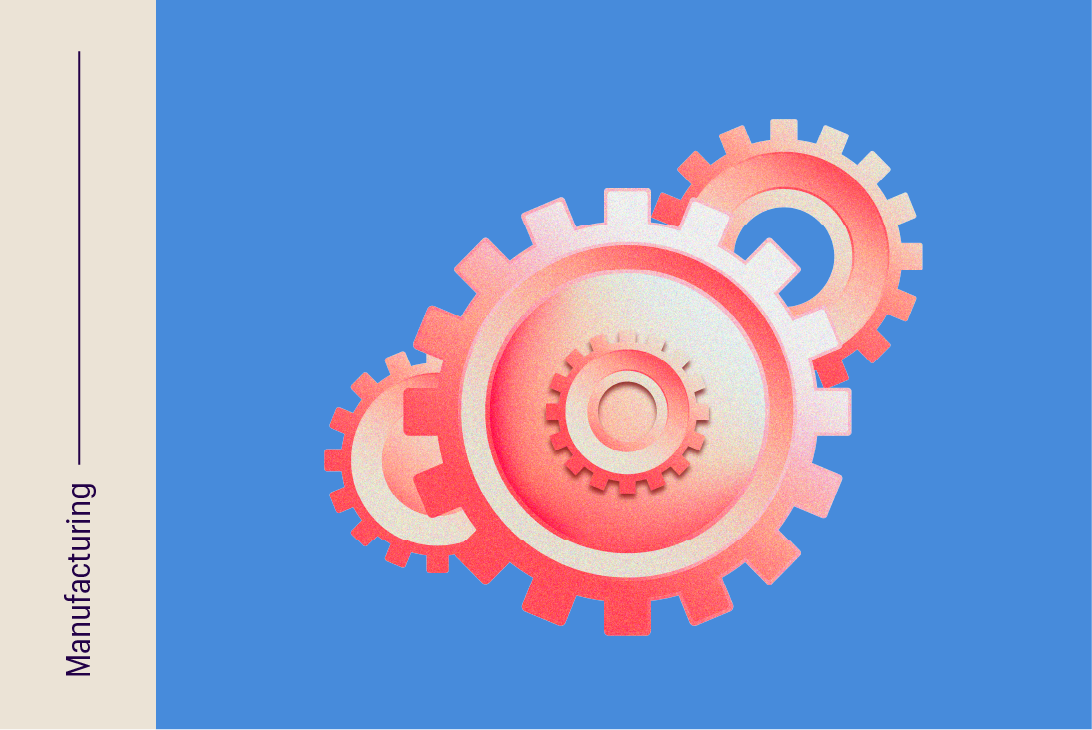
Problem statement
A leading manufacturer sought to transition from reactive maintenance to a scalable, data-driven Predictive Maintenance framework. Despite substantial investments, they faced significant challenges, including integration with legacy systems, slow adoption, and frequent disruptions. These issues not only escalated costs but also resulted in underutilized IIoT capabilities. To get the expected ROI and optimize asset performance, they needed to overcome barriers in adoption, execution, and scalability. Additionally, inefficiencies in data management and business operations further hindered their ability to streamline maintenance processes and achieve their goals.
Data Challenges:
- Inefficiencies in Data Handling: Fragmented data pipelines and slow real-time processing hindered decision-making and operational responsiveness.
- Limitations of Rule-Based Detection: Static rule-based systems miss complex patterns, leading to undetected anomalies and inefficient maintenance planning.
- Sensor Data Quality Issues: Noisy, inconsistent data streams with gaps and varying formats compromised insight quality and reliability.
Business Challenges:
- Unplanned Downtime: Reactive maintenance led to frequent disruptions, escalated costs, and underutilized IIoT investments.
- Scalability and Adoption Issues: Heavy dependency on expertise and resistance to change slowed adoption across sites.
Impact
Operational Efficiency:
- 40% reduction in critical equipment failures.
- Enhanced uptime with predictive maintenance.
Cost Savings:
- ~$1 million saved annually through optimized schedules and reduced downtimes.
Compliance & Integration:
- Strengthened adherence to industry standards.
- Seamless integration with existing IT ecosystems, ensuring minimal disruption.
Access the Case Study to Learn More about This Partnership
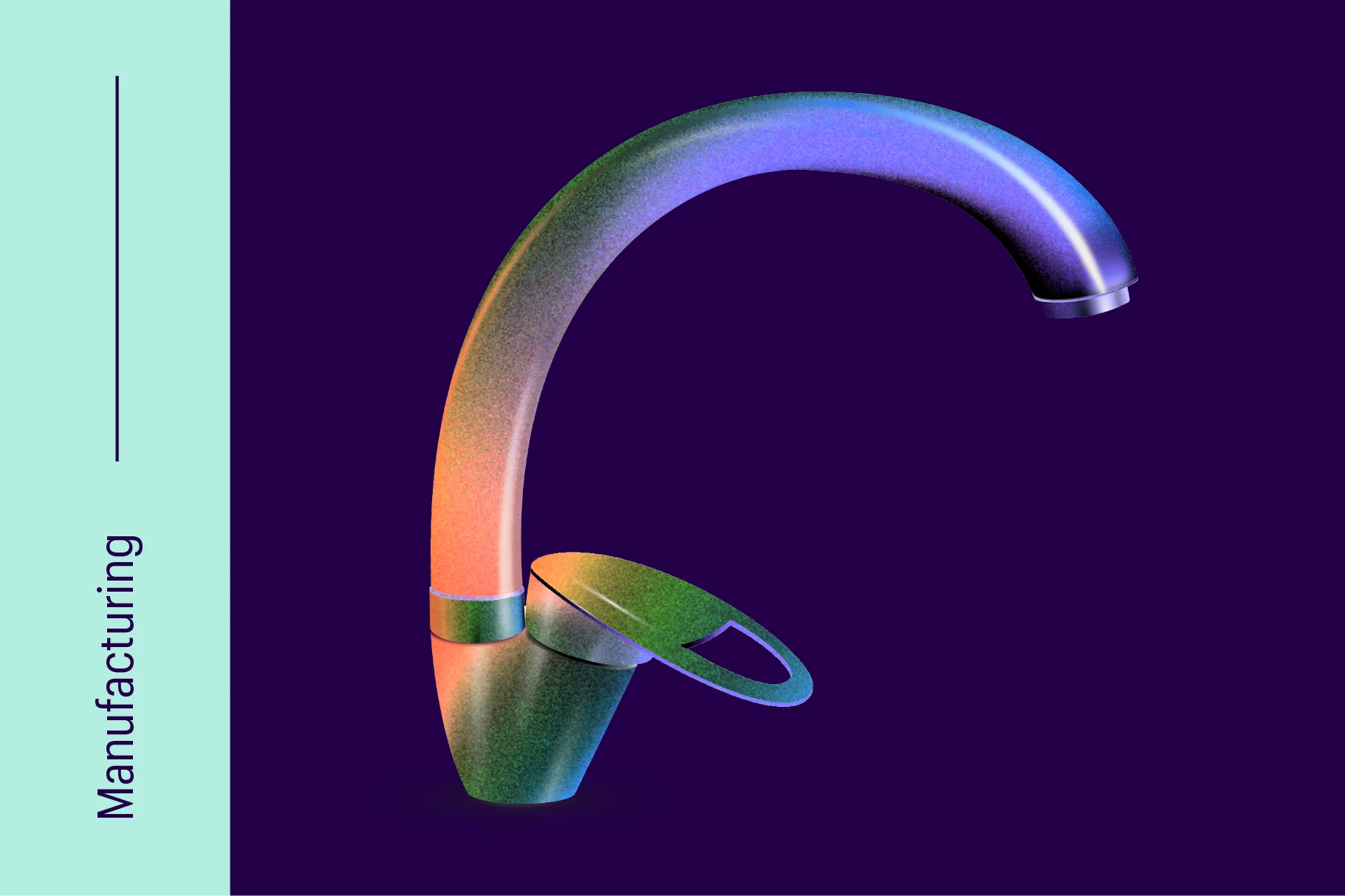
Manufacturing Demand Forecasting
We helped a leading plumbing giant discover an estimated $2 million savings in production costs using a manufacturing demand forecasting solution.
Read more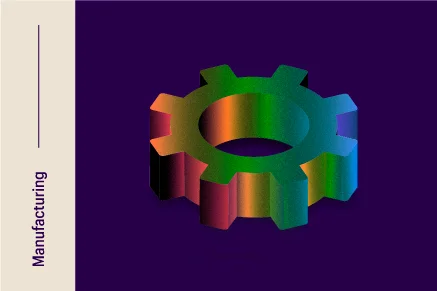
Monitoring Manufacturing Machinery
Frequent maintenance of machinery in manufacturing facilities can lead to downtime and increased expenses. By implementing real-time analysis and reporting dashboards, manufacturers can access vital data to proactively detect defects and deviations.
Read more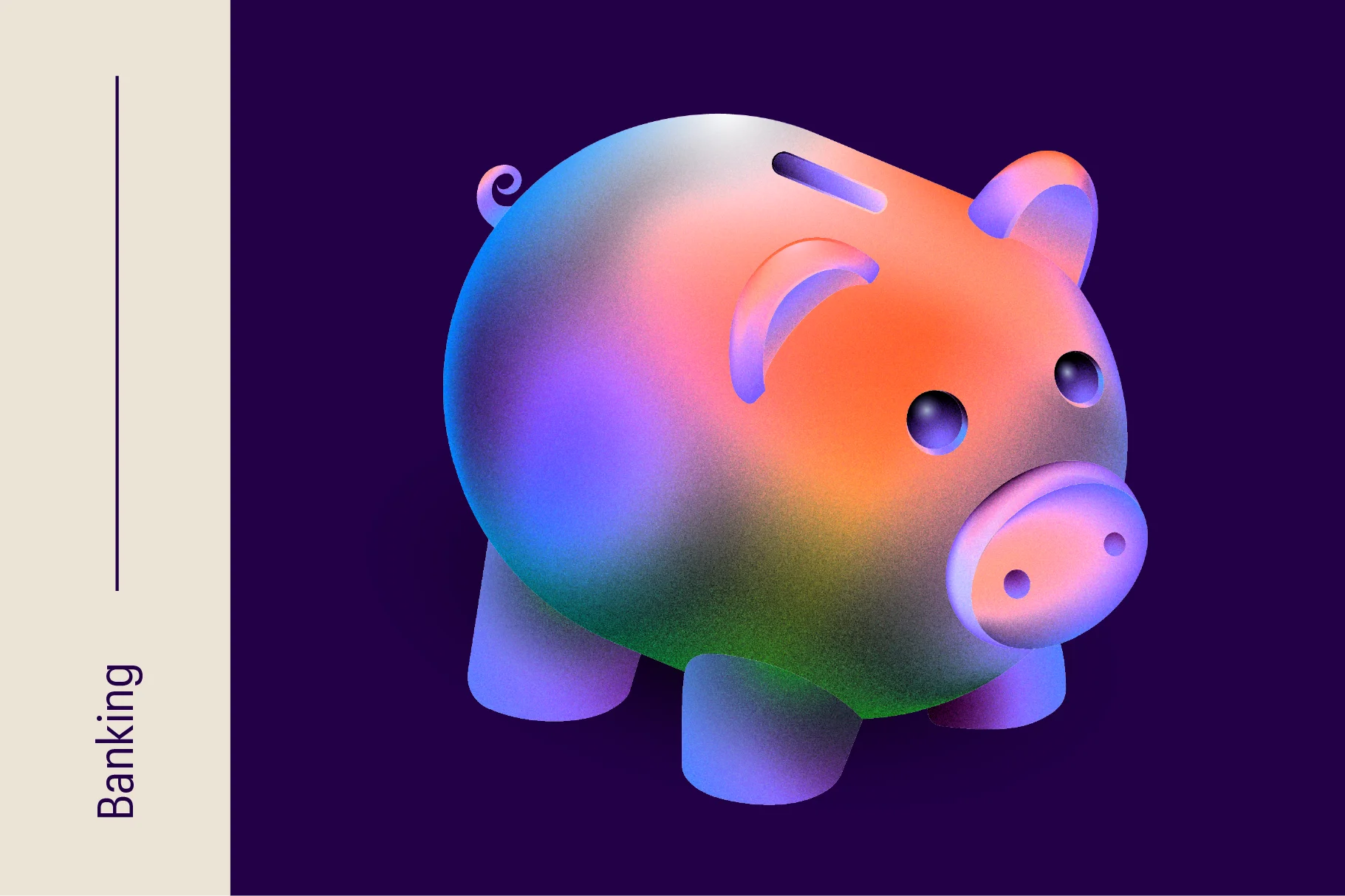
Predictive Cross-Sell Targeting
We worked with a prominent bank to create a product cross-sell recommendation engine based on key life events.
Read more