This episode is the first part of the interview with MathCo Cofounder & CEO Sayandeb Banerjee, where he talks about what it means to be a truly data-driven business.
In the episode, Sayan talks about when—in his 25 years of experience in the field—he first realized the power of data. He tells us how he disagrees with the now overused expression “data is the new oil” and shares his own analogy for the importance of data in today’s world. We discuss the history of data recording, visualization, and interpretation—in a society and business context.
He describes what a truly data-driven business would look like and breaks down the journey an enterprise typically goes through from being data-ignorant to data-driven.
He also opens up about his opinion on whether a company should build its own data analytics capabilities or depend on external vendors, and MathCo’s philosophy of promoting self-sufficiency in their clients.
You can also read the full episode transcript:
Read hereEpisode Intro by Host
You are now tuned into Coefficient, a podcast brought to you by TheMathCompany, and I’m Nirupama, a journalist, researcher and podcaster. Currently, the Content Lead for Thought Leadership and Podcasts at TheMathCompany. In each episode of Coefficient, we talk to experts, and deep dive into compelling topics related to the present and future of data analytics and AI for business intelligence.
In this first episode of the series, I got to speak with Sayandeb Banerjee, Cofounder and CEO of TheMathCompany. Sayan, along with Anuj Krishna and Aditya Kumbakonam, started the company almost seven years ago. And in these seven years, has driven MathCo from being a bootstrapped startup with a handful of employees, to a globally renowned fast-growing company that works with some of the largest brands in the world.
It’s always a pleasure to talk to Sayan. With nearly 25 years of experience in this space, he’s a treasure trove of insights. In fact, if you’re interested to know more about his journey and about what exactly we do here at TheMathCompany, and how we got here, I recommend that you listen to Sayan’s recent interview with Forbes India for their Startup Fridays podcast. I’ll add the link in the description. So we’re not delving into those details in this podcast.
For the first episode of the series, we’re starting with a topic that’s fairly basic, but incredibly crucial to understand—one that will lay the foundation and set the ground for the more specific conversations we’ll have in future episodes. We’re talking about what it means to be a truly data-driven business.
This Episode features the first part of the conversation. Let’s dive straight in.
Episode conversation
Nirupama: Hi Sayan, welcome to Coefficient, the first episode of the podcast. Very happy to have you here. So my first question to you…I’ll dive straight in, is something I’ve been really curious about for quite some time. So there is this, you know, very often used but sort of cliched phrase called data is the new oil, right? You read it everywhere, you hear it everywhere. I think for a decade people have been using that now. So I wanted to understand—do you agree with that? Or if you had to come up with a different analogy to explain how important data is for an enterprise, then what would that analogy be?
Sayan: Yeah, no, I think, thanks for asking that question right away. See data is new oil is—it was probably relevant when this term was coined. Right? So 10 years back, 15 years back. You know, for the role that data played in an enterprise, probably this was a good analogy. But if I look at today, I definitely don’t agree with this analogy, and I’ll tell you why before I go into my own analogy.
Oil is a scarce commodity. I mean, people are, you know, struggling to figure out where to get oil from. If I have control on oil, then others are in problem. But data is everywhere today. Right. I don’t think there is any scarcity of data. If, if at all, you know, the scarcity is of how do you harness the power of data?
Yeah. Talking of an analogy, I think, I don’t know if it is new something, but data is like water. And I’ll tell you why I’m saying data is like water: one, you know, data is omnipresent. It is everywhere. That is number one. Number two, just like water, you know, unless water is harnessed properly, unless it is stored—you build your dams and you build your whole irrigation system—water is of no use.
Nirupama: Yeah.
Sayan: Same happens with data, right? Data is getting generated every millisecond, every moment, every part of our life. But unless it is captured, harnessed, stored, cleaned, they’re of no use. So you see a lot of analogies with water.
And I feel that if you have to call it anything, then probably data is water. I don’t think we can call it the new water. And most importantly, it is as essential to life. Oil is very important. It fuels a lot of things. But without water, you won’t survive. I think data is getting very close to that place where without data…Now we look at any enterprise across the world; any individual human beings across the world, I don’t think they survive without data. You look at you and me, you know, kind of life without data, is a hermit life in a jungle. Right? From the time you wake up in the morning, you are using data in some way or the other. You don’t even realize.
Nirupama: Right.
Sayan: So, so that way I think it’s something omnipresent; it’s something which is very, very important. And that’s why I’ll call data as water.
Nirupama: Great. I think we are off to a great start. The next question I have for you is actually…you know, just a bit of curiosity for me to understand. You have been working in data space for, you know, ever since you started working, right? So, um, when was the first time you had this realization personally about the power of data?
Sayan: Well, that’s…you know, we have to go back, travel back in time quite a bit. So my background—I’m sure you know that I have done my Masters from Indian Statistical Institute, studied a combination of economics, mathematics, statistics, a bit of computer science.
So I have—even all the way from my college days—I have worked with data, right? In some way or the other. But obviously, you know, when you are in college, you are more interested in how to learn these things. You don’t realize the power of data, or anything like that.
In my first job with GE—and this happened in the first few months of my job—I remember we were doing a project on customer segmentation. Now, in today’s world, this is, you know, plain vanilla, everybody does that. But I’m talking of 25 years back. So using data to segment your customer base was not as prevalent.
Nirupama: Right.
Sayan: And we did that, you know, purely using data. I had no direct exposure to that business. This was a private label credit card business that GE used to run. So for one of the portfolios, we did a customer segmentation. We came up with some six, seven, you know, key segments in their customer base. And then when we were presenting it to one of the senior VPs…I remember, of that company—and that person, you know, kind of lives and breathes that business. And that person was saying, “Wow, this is amazing! Because I knew that there is a cohort of, you know, working moms who are, using this card every weekend to buy this. But I never saw any validation. And now that I have validation, I’ll go ahead and, you know, create a whole campaign and design a whole campaign to target that particular segment.” I think, and this was very, very early days, and you know, a large brand who’s talking about changing their strategy to target certain audience using the work that I’m doing using data!
So if I reflect back, I think that’s probably the first time it started clicking that that data can make a lot of difference. And then, you know, the rest is history. You know, over time I’ve had many, many different opportunities where I clearly saw impact of data in making a difference, particularly in the enterprise world.
Nirupama: That’s a great origin story, if I may call it that. Um, so next I wanted to talk about how businesses, you know, started using data. So I have learned when I was in masters…I had a research methodology class. And there was this very interesting story—I will not delve deep into the story—but there’s this very interesting story about this guy called John Snow. Um, who was the reason why people figured out that cholera is actually caused due to, I mean, it’s a water-borne disease and not just, you know, because of bad air—which is what people used to believe at that time. And that he did, because he just plotted the houses of the people who were infected, on a map of London. And he figured out that the water pipelines were sort of, you know, the pattern. And that’s how, he got to it. So that was like my moment, you know, realizing how important data is. And this is in a society context, right? So, in a business context, um, have businesses always been using data to some extent or the other to make business decisions? Or were there some sort of, you know, key moments in history, where you know, a company did this and, that was like a pioneering moment?
Sayan: I think in general, I don’t know of, in whatever I have studied and I have understood, I don’t think there is one pivotal moment that, you know, this is the time that people started using data. In fact, you give a great example. You know, I’ll go back many, many more years and, and talk about cavemen. When we used to, you know, this human race has not evolved into what it is today.
And there is enough research that says that, you know, all those cave paintings that you see. It’s not always just paintings. There is lot of data that is getting recorded there.
Nirupama: Oh, okay.
Sayan: And this could be data about, you know, the moon cycles, right? There is, you know, kind of, this is the time when then you have high tide, you have low tide. How do people, you know, keep those counts and use the data for their actual living? Because based on the tide cycle, so much can change in those days. Right? And those records were actually kept using, you know, the drawings on the caves. There is some, you know, good amount of research and I think you can look at that. Again, this is not a classic enterprise example, but the point I’m saying is that from that time onwards, human beings have been using data in different ways and, and you know, the example that you gave is great.
There are definitely some watershed moments for sure. Um, one watershed moment is, you know, when computer or computing came into being, right. That’s the first time where data started to be, you know, we can start storing data and leverage data…and the whole thing around the Turing machine and how it deciphered the ENIGMA, the code, and all of these. What is happening? So it’s all data, data patterns…But the ability to run that many iterations and not just what we are doing in our mind definitely is a shift in terms of using data. Because even if there is intent to use data, unless you have abilities to use something like at least a good calculator or a computer…you know, in your mind there are only certain things that you can do.
Nirupama: Right.
Sayan: So the invention of computer is one, you know, good pivotal moment.
Another one is, you know, is the start of database. I’m talking about early seventies, late sixties, you know, Oracle. Oracle kind of revolutionized the use of data, because that’s probably one of the earlier instances where the whole concept of database came into being, right?
And, and we all know that, you know, the data is useful when we start storing data, and look at historical trends. Because the data which is available right now only gives you a certain story. The history is important; what happened before is important. So that is another, you know, very important moment. And then, then obviously this whole internet.
Nirupama: Yeah.
Sayan: I mean, when you know, things started getting connected. The worldwide web, as we call it. That’s another big watershed moment because now you not only can use your own data, but you have access to others’ data in some way or the other. That’s another big thing. So there are some watershed movements. Some moments where the velocity or, or the way the data is being used, obviously grew leaps and bounds. But, but it’s still a very, you know, kind of an incremental, kind of a line, with some blips. It’s not like, you know, it was going straight and then suddenly went up. So there was no one really magical moment. But there are definitely events that made a big impact in use of data.
Nirupama: Right. So now we’ll get to the main topic of the conversation. So the topic we’re talking about today is, what it means to be a truly data-driven business, right? So, to get started on that conversation, could you describe to me what a truly data-driven business would be? Like, if you could describe it.
Sayan: Sure. Um, look, I will give you, you know, my interpretation of what I understand, and what I think a lot of people that I talk to understand as a truly data-driven organization.
A truly data-driven organization is one—I’ll first use a simple definition and then we’ll break it down to see what that means—is an organization who is using data or evidence to make every single decision that they need to make.
Okay, so now, now let me break it down. So if you look at any enterprise, any business, there is an external facing side of the business. If there is a manufacturing company, they’re manufacturing certain products, certain goods, and then they’re selling it to the market. Use of data on the external side, I think is now still pretty well-established…You know, how many units do I need to produce? Who are typically my buyers? What price do I sell it at? Use data for making those decisions. Even there, you know, there are different levels of maturity. There are some who are right up there, some are not there.
But I think what is more critical for an organization to be, to become truly data-driven, is when we look at internal processes, internal decision-making, and use data for that. So what I mean by that is…The number of people or different kinds of resources that are needed by an organization is determined by, you know, data, data trends, and data patterns. Individual productivity is determined by looking at data and figuring out where I’m very productive, where I’m not productive, right? Operational efficiency, which is very internal focused. Understanding human performance, you know, who is doing great, who is not. So, each of those small and big decisions, if they are driven by data…And I call it evidence—you know, when you say data, there’s a lot of questions. There is at least some evidence that because ABC happened, therefore this will happen. That’s when an organization becomes truly data-driven. And if you now look at that organization from outside, if you have a bird’s eye view, you’ll see that every interaction that is happening within that organization, as well as when the organization is interacting with the outside world…So all external interactions and all internal interactions—there is a data component in that conversation. There is data, which is…think of this as a blackboard behind where the data is being projected and the conversation is happening in the context of that data point.
And this is all internal one-on-one, one on many, you know, different kind of conversations as well as external, both. And I started by saying that while there are many today who have matured on the external side to be leveraging data, leveraging it for internal, purposes is something which only few do and do well.
And that’s what a truly data-driven organization is.
Nirupama: Right. Now that we’ve set this context, I want to delve a little deeper into this. Um, so to me, it’s obvious that a company doesn’t, you know, directly go from being, let’s say, data ignorant to being data-driven in one day, right? So, um, are there any recognizable stages or phases, in which a company goes through this journey?
Sayan: Sure. See, there are many ways. And first of all, you’re right. I mean, it, it doesn’t happen overnight. It’s a journey. Some, you know, traverse through that journey much faster and some take much longer. And it depends on hundred different factors. One, one very popular way of breaking down these phases is…there are four phases to it.
So, so today I’m data ignorant. I’m not leveraging data. The first step is to say that, can I use data to see what happened? So, use data as just a rear-view mirror. Okay, so I’m an enterprise. Think of this as a driving the car. Can I at least see, you know, what I have crossed, what I’ve left behind? This is what is called a descriptive phase. Okay. So there are many organizations even today who do a lot of good descriptive work. And probably they have not made progress beyond descriptive as much as they aspired to.
The second is what is called inquisitive. Inquisitive is nothing but why something happened. So while I am driving, through the rear-view mirror, I’m seeing that I have crossed this milestone. I’ve done this, but I also realize that I have slowed down for the last one hour while I’m driving. Now the question that, why have I slowed down? Is it because the road conditions are bad or because I am little fatigued so I’m not driving it right? So in the business world, I mean, I have run these three campaigns. I know in the descriptive side how they have performed, but on the inquisitive side, you start now talking about why something happened. Root cause, right? Again, this may sound very simplistic and very fundamental, but believe me, it doesn’t happen that easily. So that’s one big leap. Because now people are saying that, okay, I’m trying to learn something from data so that I can make my decision better.
The next step is, we call it predictive. I’ve learned what has happened, I’ve figured out why something has happened, but now a very important question, what will happen next? So I’m not just driving and figuring out what I have left behind and why I have slowed down. Question is, will I completely stop in the next few minutes or will the car just come to a crashing halt? Or will things change? So, predicting what will happen in the future by using all the knowledge of…And by the way, these are all incremental. So just because somebody is inquisitive doesn’t means that I don’t need descriptive.
Nirupma: Descriptive, yeah.
Sayan: Descriptive becomes a fundamental, the baseline, right? So then you build inquisitive on that. Now you build something on top of that, right? Which is predictive. That’s where I think in one way it also starts becoming a little more data-driven because I’ve not only learned what has happened, but I’m trying to predict what will happen tomorrow. What the demand for my product will be, not what it has been in the past, what it will be based on macroeconomic scenarios, you know, what competitors are doing, my pricing strategy. So many different things, right? So what will happen tomorrow is a very important question that needs to get answered. That’s called predictive.
And then finally what they call as prescriptive is: ‘So what?’ You know now that you have learned what has happened, why something happened, what will happen tomorrow, what are you going to do about it? Because that’s when you know what you’re going to do about it—the decision-making part of it.
Nirupama: Yeah.
Sayan: And that’s really the, at least somewhere, the nirvana where, you know, I am figuring out or I’m deciding on what decision to make based on what I’ve learned from data, what has happened, why something happened. So this is one way to look at, you know, the evolution of a company or an enterprise from being data ignorant to really leveraging data and data-driven, to some extent.
Nirupama: Right. Thank you for taking all the listeners through that. Um, another thing that, you know, I can sort of guess from what you have shared is…As far as I can see, there seem to be two paths to take in this journey. One is, you know, you work with experts, external experts who will do this for you. Or the other is, you know, you invest in these capabilities. You know, in the personnel and infrastructure so that you can do it yourself. So how does a company decide which path to take?
Sayan: So it’s a great question. Um, I’m sure I will say something which, you know, some will agree with, some will not agree with, but that’s how the world is. But I’ll only talk about, you know, what I have seen over this last 25 years, what my experience had been.
Nirupama: Right.
Sayan: Uh, first things first, I don’t think it’s a question of either or.
Nirupama: Okay.
Sayan: Uh, I think. You know, there is an English word, a three-letter word called “and”. Which is, which is very, very powerful as they call it—the power of “and”, right?
So it is about building internal capabilities, becoming more and more self-sufficient, and leveraging partners for very specific purpose, AND…but let me, let me illustrate that a little more.
I think, in my experience, what I’ve seen is organizations go through this journey. So when they start, and I just described, when somebody goes from being data ignorant, they start doing descriptive work and inquisitive work, I think initially—and naturally so—there is a dependence on a partner, to do this, to bring this kind of insights to the table, to bring this ability to the table because, a partner who has been doing this, leveraging data, and understands that industry’s dynamics, right? So they come in.
As we get more and more matured, and we go through that cycle—descriptive, inquisitive, slowly becoming predictive…What I have seen is, there is an increasing realization that, “Hey, while I am working with a partner, I’m not building any IP on my own.” So you see there is a shift. Slowly, there is a shift from dependence to independence: that I’ll be independent, right? Which is great. And I strongly believe that any enterprise needs to have a certain amount of independence and self-sufficiency as far as data and data-related abilities are concerned. Because as I said in the beginning, you know, this is like water. You have to figure out how to harness water yourself. You cannot be dependent on the canned waters coming to you and just drinking that bottled water. You have to figure out ways to clean water, you know, kind of how to use this better. It is very important in the journey of an enterprise.
However, I think as they become even more matured, you’ll see that from dependence to independence, it starts becoming an interdependent relationship.
Nirupama: Okay.
Sayan: So what that means is that, you know, I definitely want to have my independence—I’m talking from an enterprise standpoint—but I am, you know, kind of smart enough to know where to leverage a partner.
So I’m dependent on them in certain aspects. The partners are obviously dependent on me to, you know… So there’s a relationship of interdependence that also starts growing.
And this can be in many different areas. See there are newer areas that an enterprise wants to, you know, explore with data. Typically, that journey starts with a partner because you know, who knows how successful that would be? If I’m exploring a new way of looking at customer acquisition, leveraging data, right? So I will try for a bit, but unless you know you have tried that enough and you see success, you don’t want to build your own capabilities because you don’t know that in, you know, six months, nine months or a year’s time, maybe you have to change track. So that’s one area that I’ve seen, you know, people leveraging a partner.
People talk about change is the only constant, right? So with that change, it means that, you know, there is new technology, there is a new methodology, there are new ways of looking at data/leveraging data to make decisions in a very different way. Enterprises are very cognizant of the fact that all of that cannot happen internally, no matter how much I invest in research and development. Because at the end of the day, data is not their business. Their business is still, you know, production of a certain commodity or you know, kind of providing a certain service, right? Or running a bank or, you know, running an insurance company. So those newer capabilities and newer things have to come from outside.
So there are few, you know, very established ways in which I have seen, as organizations go through that maturity, they use the power of “and”. And, you know, I, and we in TheMathCompany, have been a big believer that organizations needs to have a certain amount of self-sufficiency. Only then there could be a very healthy engagement and relationship with an external partner. Otherwise, you know, there will always be a little bit of that mistrust that, oh, I’m a hundred percent dependent on them, and there is a fear that, you know, what am I missing out on?
So that way I have honestly, um, you know, advised many of our clients that you know, “I don’t think you have the right internal capability today. Why don’t you also build your internal capability while we work with you to solve problems A, B, C.”
Nirupama: Okay. Um, so I, uh, just want to branch off and ask a follow up question from that.
Sayan: Sure.
Nirupama: You know, to a listener, I’m a little curious, right? As a company that provides these kind of services, you know, encouraging your clients to become self-sufficient—isn’t that counterintuitive?
Sayan: No, it is not counterintuitive and I’ll tell you why. I think it is counterintuitive if you come from the fundamental belief that there is a finite number of problems that need to get solved.
Nirupama: Okay.
Sayan: And if I am helping my clients or these enterprises to become self-sufficient in solving those problems, then there is nothing left for me to do, right? It is coming from that fundamental hypothesis. The reality in the world today is that the demand for solving problems using data far outweighs any supply that you can think of.
So even if an organization build their internal capabilities, they can only, you know, kind of cover only a certain percentage of those problems that are out there. And by the way, this is, I’m talking about identified demand—I mean known problems. There is a lot of latent problems or latent demand that we have not even explored. So that needs to be explored. In my mind, for that there will be a role for a partner like us to work alongside enterprises to explore that.
On the contrary, you know, if I encourage them to become more self-sufficient, I think the relationship becomes very healthy. Because the client knows that we are not here to charge what is typically called an economic rent. It’s not that I’m here to do the same exercise again and again, and not let them take benefit of the IP that is created.
So I think there’s a tremendous amount of trust that gets built in this approach. And this will be the case in the near foreseeable future. That the number of problems that needs to get solved—the demand far outweighs, you know, any form of supply.
Nirupama: Right.
Sayan: So I don’t think we need to worry about that.
And that’s the macro, overall picture. Now there may be small pockets where this may not work out. But so be it, right? I mean, that’s, you know, the overall philosophy with which we are building TheMathCompany.
Episode Outro by Host
Thank you for listening to the first Episode of Coefficient. As I mentioned at the start of the episode, this was just the first part of my conversation with Sayan. Don’t forget to tune in to listen to the second part where we discuss the cultural and organizational changes that are important in a company’s journey to becoming data-driven, the most common mistakes companies make in this process, among other things. Next episode is launching later this month—do subscribe to the podcast to be alerted when it’s released. Goodbye and have a nice day.
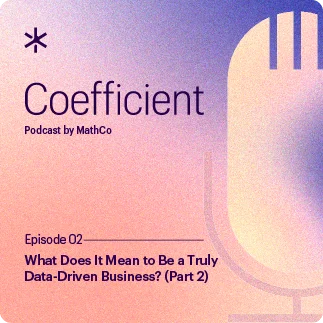
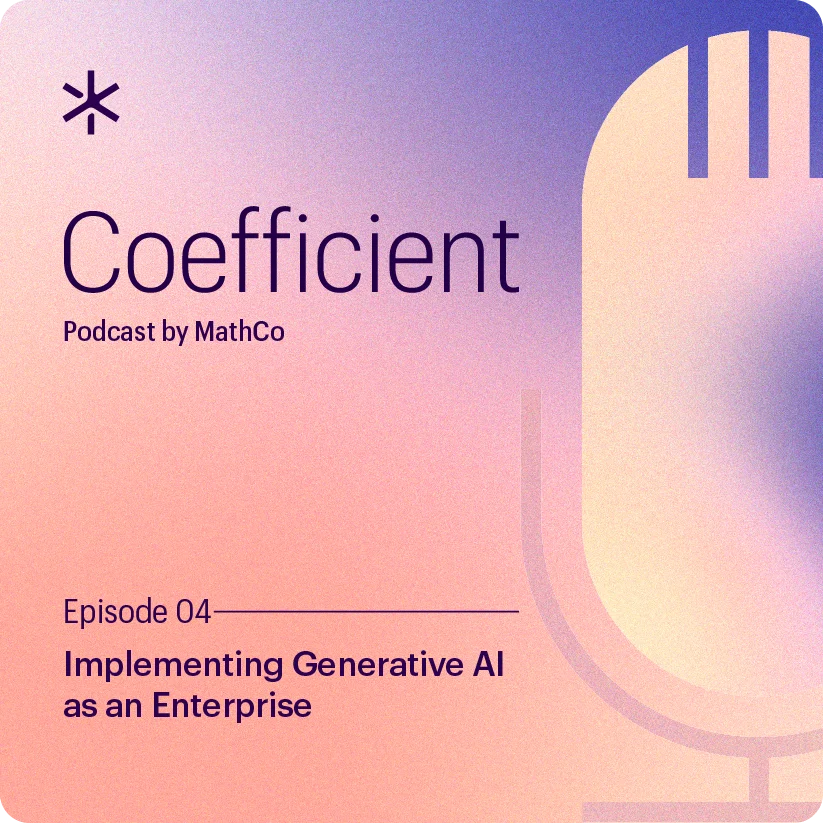