This is the fourth part in an on-going series of Assessing Analytical Maturity – Are your analytics efforts viable? If you are new to this series, we recommend reading the first part here, the second part here, and the third part here.
In our quest to assess analytical maturity of an organization, we have covered ‘data as the new oil’, and talked about ensuring a good infrastructure to mine, refine, and generate value from it. We take our next step by assessing what is arguably the most critical aspect. The people. The talent that truly helps organizations embark on their journey of analytical transformations.
To do so, we will attempt to cover the vast extent of this cog in the wheel of assessing analytical maturity in as extensive a way as possible.
Consequentially, this part has been split to cover the following:
- What are the roles required to build great analytical teams? What are the skills needed?
- How do the various roles interact with each other during any solution development?
- What are the analytical teams of the future going to look like?
- How does hiring keep up with the ever-changing needs to build the analytical teams of the future?
- What role does training, learning, and development play in helping the employees chart out a great career path?
- And finally, what are the other factors that play a role in ensuring a low employee attrition rate?
In one of our earlier posts, we have touched upon the roles data analytics teams of the future would require impacting an organization’ analytics maturity curve. As an amalgamation of algorithm development aided by domain knowledge, supplemented by contextual understanding, and requiring a skill in coding, analytics teams have historically been staffed by people expected to play a wide array of roles akin to a Swiss Army Knife.
An analytically mature organization understands the need for specialization. Great teams employ and then deploy people bringing in a specific skillset to play a specific role. The various roles that exist within analytics teams can be visualized thus
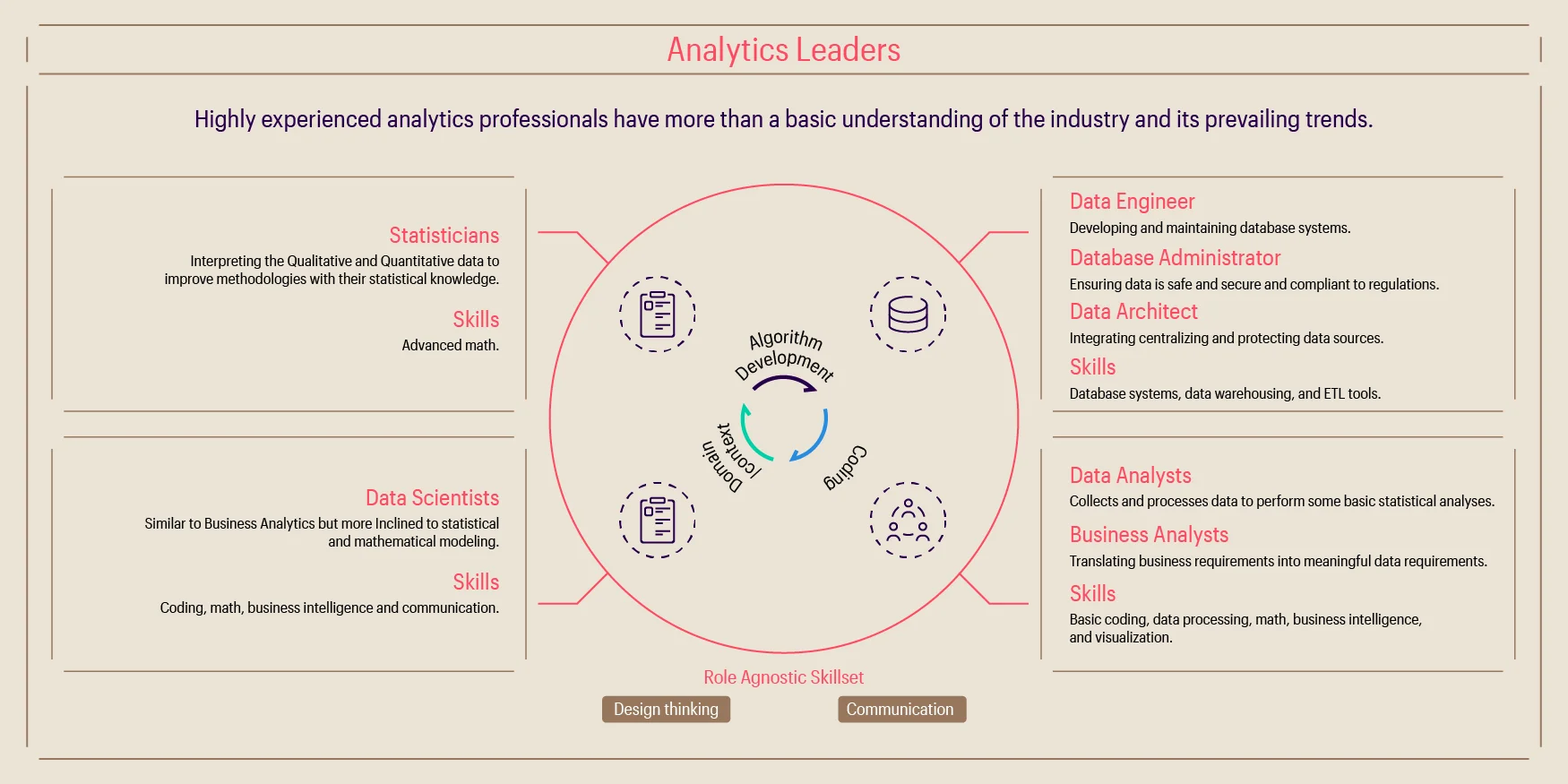
Roles in analytics teams that drive organizations’ analytical maturity:
Analytics Leaders are highly experienced individuals and shoulder multiple responsibilities. From setting up the analytics team to defining the ‘Why’ of the problem to envisioning its impact to providing guidance during solution development to evangelizing the solution across the functions, Analytics Leaders are charged with building a culture within the organization that is analytically driven and does not limit itself to using analytics to augment decision-making. With their understanding of multiple domains, they can link and learn from one industry and apply it to another, thereby casting a significant impact on organizations’ analytics maturity curve. Their understanding of the prevailing industry trends allows them to remain ahead of the times. Any analytical transformation journey has these Analytics Leaders as its lynch pin.
Roles requiring an element of coding and an understanding of data engineering bring the Data Architects, Database Administrators, Data Engineers, and Product Managers together. With an extensive knowledge of the database systems, data warehousing, and ETL tools, the people in these roles are responsible for designing the data architecture, integrating, and centralizing the data sources, developing and maintaining database systems all while ensuring that it is compliant with the prevailing law of the land. Product Managers ensure the final solution can be socialized with business stakeholders in a productized form.
At the cusp of data, data engineering, and business domain end of things lie the Business Analysts and Data Analysts. With their high acumen to translate the business requirements into meaningful data requirements, business analysts help translate the business problem to an analytical problem, thereby catering to organizations’ analytical maturity. Aided by data analysts who collect and process data in addition to performing some basic statistical checks, the problems are defined better, hypotheses are generated, and the final solution is visualized in a manner fit for consumption by business stakeholders.
Data Scientists are charged with applying the domain understanding (aided by the contextual understanding) to the various algorithms. With a profile and skillset largely similar to business analysts, data scientists are slightly more inclined to statistical and mathematical modelling. Coding, math and algorithm development, and an understanding of the business form the core of what is touted as ‘The Sexiest Job of the 21st Century’ [1]. Data scientists follow an iterative approach, implementing a multitude of methodologies and algorithms to solve analytical problems.
And finally, we have the Statisticians who apply their advanced statistical knowledge to interpret qualitative and quantitative data. They improve the existing methodologies with their statistical knowledge and help provide heuristic and statistical methods to test various hypotheses and drive organizations ahead in their analytics maturity curve.
The skillset of Design Thinking and Communication is role agnostic and underpins them all. To be able to think in a logical and systematic manner and communicate effectively with the other members of the team ensures smooth transition and successful execution of any analytical problem.
How do these roles interact during a solution development?
As mentioned before, a successful team and an analytically mature organization ensures there is a good mix of the people to develop analytical solutions. And they play a vital part at every stage of it. A summary of the above roles during various stages of solution development may be visualized as
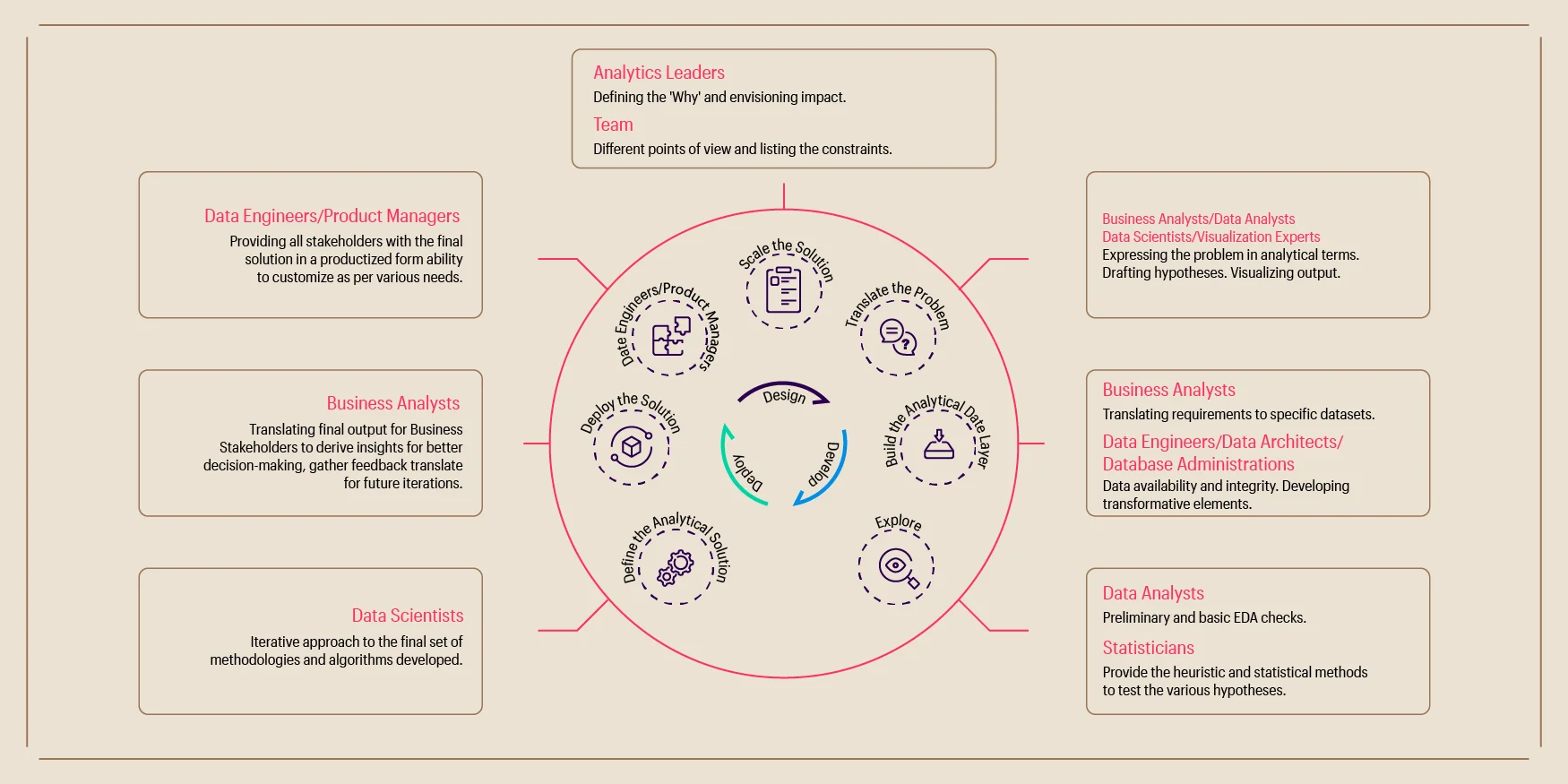
What are the analytical teams of the future going to look like?
Organizations are always on the lookout for being ahead of the times that will be defined customers’ evolving demand. For instance, clients demand for the complete ownership of AI solutions that they procure. This necessitates the inclusion of people with specialization on futuristic technologies such contextual AI. The roles for such teams have been covered in our earlier post. However, to quickly summarize some of the roles that will become more prominent:
- Software Developers – Developers and testers creating an offering that is on a more user-friendly platform
- Data Collection Experts – Ensuring the data collection is moved from an ad hoc manner to a more streamlined and scientific manner to maintain its accuracy
- Requirement Engineers – Articulating the subtle customer needs to be based on the ever-changing demands and business circumstances to strategize concrete plans for delivery of advanced solutions such as contextual AI in a more comprehensive and implementable manner
- UI/UX Engineers – Ensuring a greater business consumption by designing a platform and/or visualizing the output for a better user experience
- Compliance Liaisons – Keeping up with the stringent data security laws require these specialists to play a pivotal role
- Ethicists – Bringing a moral lens to the aggressive data collection and its ethical usage will require ethicists thereby averting largescale PR nightmares while understanding customer sensitivities better
We have attempted to help you assess analytical maturity based on the various roles that exist, their interactions during solution development, and looked at what the future holds for us. To understand how analytically mature organizations differ from those who are just about to embark on this journey, we need to take a deeper look into the hiring practices, followed by ensuring that the talent pool is retained with effective training, learning, and development programs. Stay tuned for more.
Bibliography:
1. Davenport, Thomas H., and DJ Patil. “Data Scientist: The Sexiest Job of the 21st Century.” Harvard Business Review, October 19, 2012. https://hbr.org/2012/10/data-scientist-the-sexiest-job-of-the-21st-century.