What’s common between a successful football team, a symphony orchestra and a blockbuster movie? What is it that makes them special? Each is made up of high-caliber individuals, who are specialists in their job. And on any given day can perform to the best of their abilities. But what makes them tick as a team? It’s the manager, the orchestrator, the director. Without them, these individuals will just be that: Individuals.
Take the example of an orchestra. The orchestration conceptualizes the theme, shapes it in reality and interweaves the individual styles and strengths of each musical instrument and musician. Even in an orchestra which has the best musicians, it takes a firm hand from a vantage point to produce fantastic music as a group. Without the orchestration, each musician may fail to channelize their individual effort into a collective performance that is worthy of appreciation.
Just like the director or orchestrator binds the team together in pursuit of a unified dream, the same way Analytics Governance is meant to steer the analytics unit towards consumption focused initiatives.
Governance is to the Analytics setup in organizations, what orchestration is to a symphony orchestra
So, in a nutshell, what does Analytics Governance achieve?
- It translates the organizational mission into tangible analytical goals
- It brings together the creators and consumers of data and analytics in the organization and establishes clear roles and responsibilities
- It understands what the consumers really need and effectively translates it to the creators
- Along the way, it educates the creators on the best practices and improves access to the knowledge and tools required
- It synchronizes the functioning of all the moving parts and reduces inefficiencies and struggles
It ultimately enables more consumers in driving better data-driven decisions
Organizations in mature industries that strive to differentiate their services and products from their competitors’ have identified that their uniqueness lies in the business processes they use for value creation. Analytics, identified now as a necessity by most organizations, needs a similar structural transformation to remain relevant within the organization. Some functions dabbling in analytics in silos may add incremental value but will not help organizations be the best-in-class.
Many leading organizations have had their own unique and challenging journeys in improving the Data and Analytics structure. They have had to juggle a plethora of factors to get the right data, people, process and technology aligned in a way that drives maximum value for their businesses.
In P&G, multiple teams funded their own analytics practice. In this decentralized analytics structure, analysts improved business processes and saved the firm money; but because they were squirrelling away in dispersed domains, many executives did not know what services they offered or how effective they could be
In KeyBank, each line of business had its own analytics group: 13 analytics teams and 13 data warehouses leading to multiple versions of the truth, fiefdoms in the organization. Moreover, there was no real career path in the analytics groups as they reported to Product and Marketing people. The business side didn’t understand what they were working on or who had asked them to do it; the analytics team wasn’t able to provide proactive consulting
In the new digital space, AirBnB started out with a centralized analytics team in 2010. But partner teams didn’t fully understand how to interact with them, and the data scientists didn’t have full context of what they were meant to solve or how to make it actionable. Over time they became back office support instead of consultants and, as a result, their work became highly reactive
Three out of the Top five pain points stated by organizations in a recent EnY-Forbes Insights Analytics Survey call for a top-down sponsorship and support for analytics. C-Level executives have realized that there is great merit in prioritizing analytics as a core organizational function akin to Marketing, HR or Finance. Analytics, having developed in a very tactical fashion till now, requires this strategic oversight.
This is where an analytics governance model steps in.
“..the most significant obstacle to achieving data effectiveness is designing the right organizational structure..” McKinsey 2016
There are 2 broad questions which organizations need to ask to design a customized analytics governance model
1. Analytics governance- leadership: who should design the analytics strategy and facilitate its funding?
In 2013, a large proportion of organizations either had a Business Unit or a Division head as their Head of Analytics, or didn’t have a single executive leading the unit. But this is slowly changing as more organizations require the C-level oversight to maximise value from analytics. In 2016, 51% of organizations’ C-suite executives fully supported the Data and Analytics Strategy and 25% stated that their CEOs lead it. (Gartner). Some other C-level designations of analytics leaders are: CIO, COO, CTO, CFO, CMO, CSO. This is a natural extension of responsibilities for these executives who oversee all the business units
In leading organizations, CEOs are three times more likely to directly sponsor the analytics initiatives whereas it is done by the CXO-2 level leaders in laggards
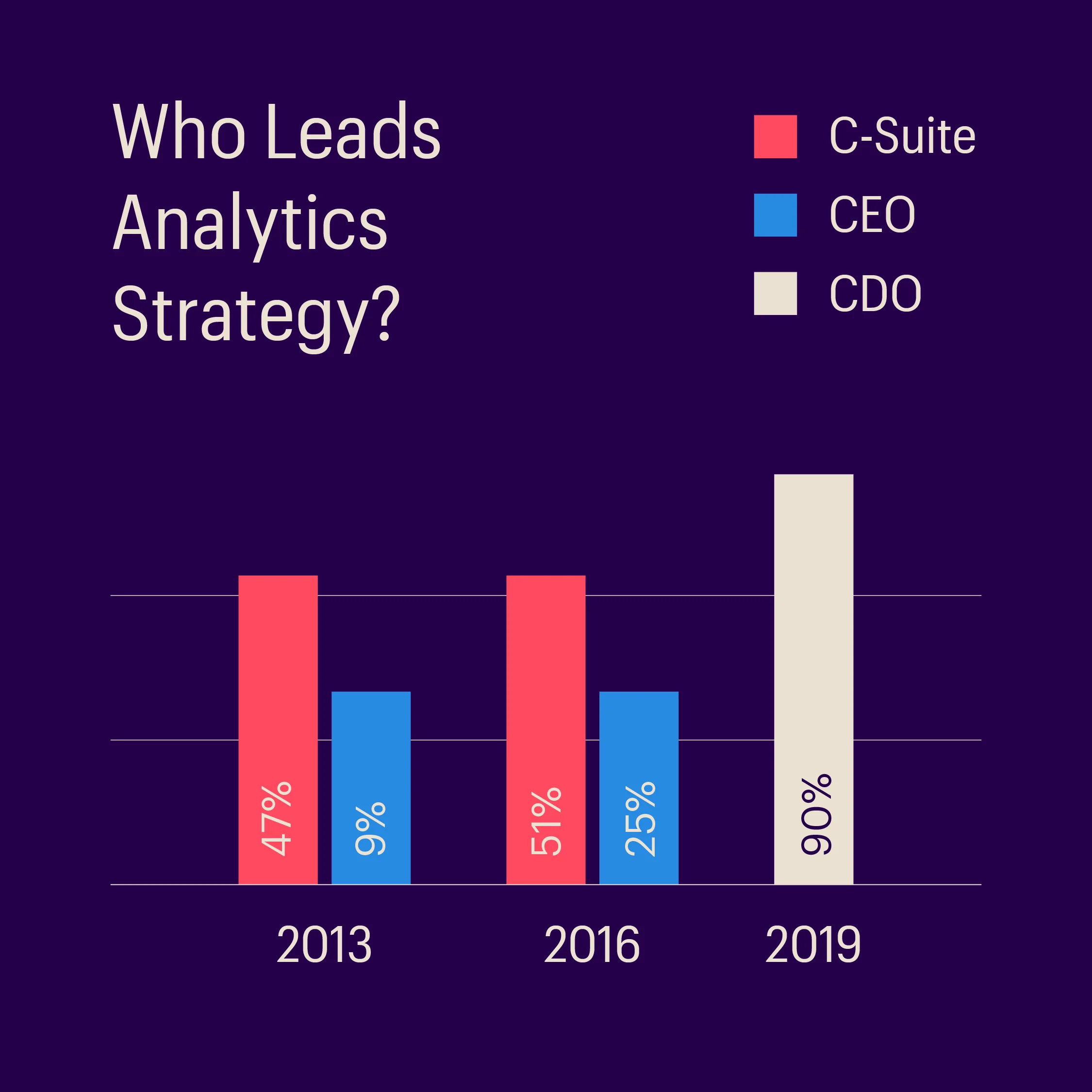
Amy Brady is the chief information officer (CIO) of KeyBank – leading the company’s Technology and Operations organization. In her role, Brady oversees all of Key’s shared services for technology, operations, data, servicing, security and procurement. The analytics CoE has about 40 people focused on analytics and another 40 on more general work such as reports and running campaigns to leverage analytics
Today, several organizations are in the process of creating new highly-specialized roles to lead their Analytics efforts.
By 2019, 90% of all global enterprises are set to have a Chief Data Officer (CDO), who will act as the authority on all data, its management and governance. Some organizations have appointed a Chief Analytics Officer (CAO), who works closely with the CDO to design and deliver analytics insights to the business. The two roles are also often combined to Chief Data and Analytics Officer (CDAO). Given the recency of these roles, the responsilibities associated with them will likely evolve in the coming years. HSBC, P&G, Wells Fargo, Ford, Cisco, Visa, Amex, IBM, P&G, Honeywell are examples of organizations who have such roles
Mark Clare is the Global Head of Data and Information Management of HSBC‘s Retail Banking and Wealth Management (RBWM) business. His responsibilities include the global integration of data management and big data into core business functions and strategies. In addition, Mark has Chief Data Officer responsibilities supporting data governance and foundation functions. Prior to joining HSBC, he served as the Chief Data Officer at JP Morgan Chase
2. Analytics governance-set-up: what is the right reporting and operational structure?
75% of leading organizations possess an enterprise-wide analytics strategy while this is true for only 1% of laggards
Centers of Excellence (CoE) are hybrid organizational structures, with matrix reporting lines between the analytics function and the business units. It provides the organization with a centralized enterprise-wide data and analytics management system, while the operational work is decentralized. Post the initial funding to set up the center, business units are charged on a fixed fee, time-based or a milestone-based pricing model, among others. This ensures balance between unit level imperatives and the central strategy. It can also lead to better consumption of solutions due to its direct reporting to a CXO. However, it is expensive and time-consuming and requires a strong top-down commitment to improve the consumption of analytics.
In the Functional Model, organizations place their analytics division under the purview of their core function. For instance, in the BFSI domain, analytics is placed within Finance. AIG has established a Risk Engineering and Analytics Center which leads its analytics practice. The next most common champion of analytics is the Marketing function. It hosts a critical mass of data and analytics practices in Retail and Digital Technology companies. Core Technology organizations have placed analytics under the purview of IT. While this model ensures that the organization plays to its strengths, the analytics reach across all the organization’s functions could be compromised.
The Decentralized Model prescribes hosting an analytics setup within each business unit. This model works well where each business unit is significantly different, has distinct data sets and can make its own data-driven decisions with limited coordination. A great example is Google, which provides significant control to Business units who make their own decisions but collaborate on selected initiatives. AT&T and Zynga are other examples.
Some mature organizations have Embedded Analytics practice into their day-to-day functioning, enabled by ‘agile governance’ which provides an environment to focus on outcomes and speed whilst reducing the red tape involved with hierarchies.
Amazon, for example has created a culture of analytics in the organization called the “Culture of Metrics”. It has setup small cross functional teams that have a mix of managers, SMEs and analysts. The teams have access to each other’s reports, which leads to the creation of a cross-functional data repository. Independent KPIs are defined for each team which are continuously tracked while making decisions.
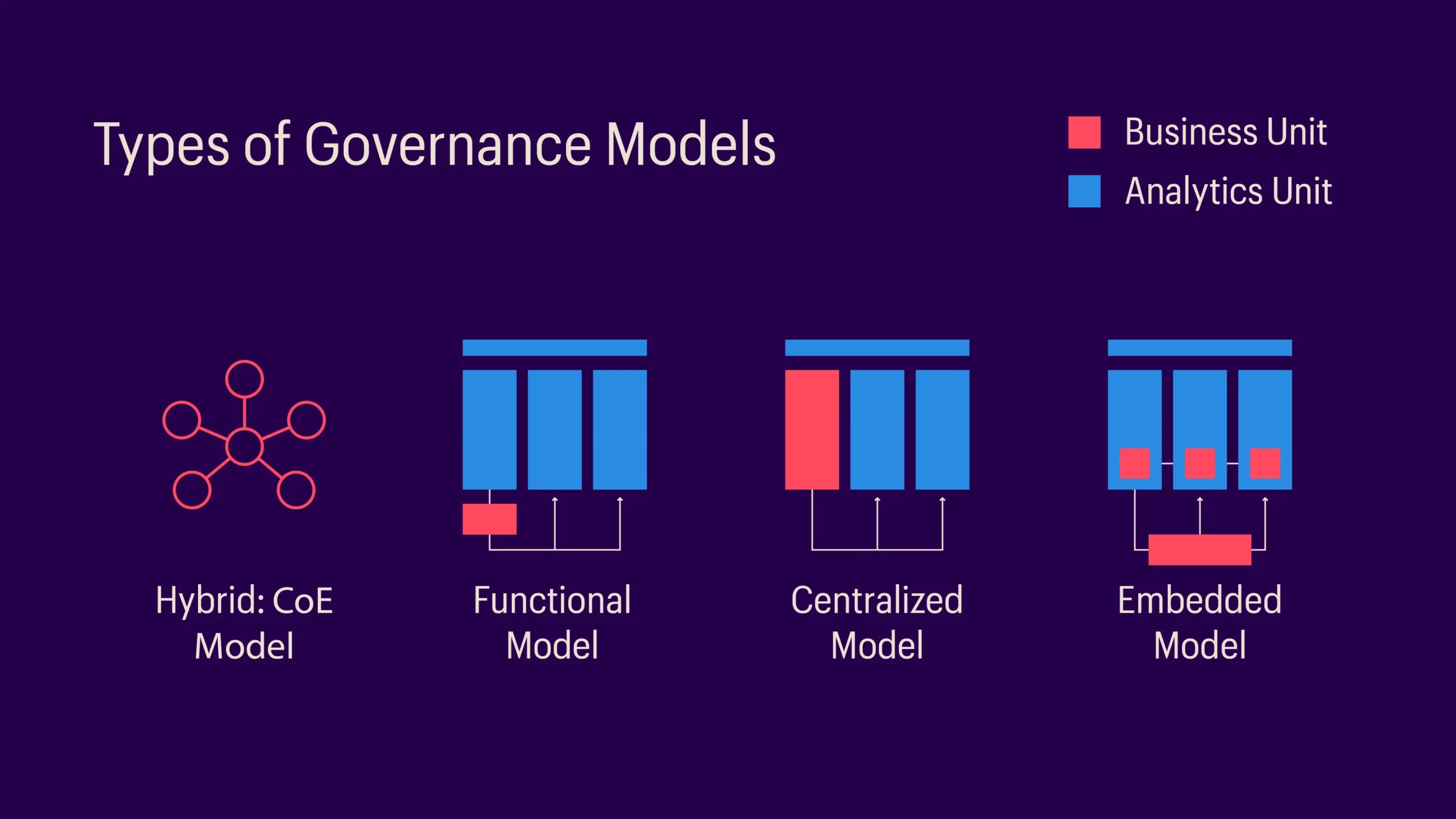
So how should organizations go about setting this up the right way?
A robust, successful analytics function encompasses more than a stack of technologies, or a few people isolated on one floor of the building. It should be an integral part of the organization, involved in all key decisions of the ecosystem. But, where it sits and who leads it is something for organizations to plan for.
Unfortunately, there is no “one-solution-fits-all” for setting up Analytics Governance within an organization. Taking stock of how things stand right now via a gap assessment is a good start for an effective analytics governance model. Narrow down on a setup that helps the adoption of an enterprise-level perspective while maintaining the right balance between functional goals and analytics solutions. Or you can learn from your success stories. Analyze the success of a function with proven analytics expertise. A function which is led by an executive who is well versed with the talent, technology and processes required to mould the analytics practice, who has demonstrated the value of analytics and is well connected and trusted by leaders across other functions.
However, before we put it on a pedestal and crown it king, one thing should be clear. Analytics Governance might be an answer to your analytics conundrum, but it’s NOT THE ONLY answer. It’s a piece of a much larger puzzle. A puzzle which has multiple complex layers and takes consistent effort to solve.