Gartner predicts that 90 per cent of business strategies will explicitly feature information as a critical asset, with analytics as a necessary competency by 2022. More and more CDOs are discovering that the right and prompt analytics training interventions can thrust their analytics functions forward, by years. But with a multitude of training approaches abound, you’ll often hear questions like, does a data science bootcamp suffice or is a real-time hands-on experience what my team really needs to speed up on the learning curve? To gain a better understanding of the kind of training that analytics entails, let’s start with the basics, by diving into philosophies of education that have been prevalent for centuries now.
The history of education:
Many philosophies of education have risen over time, owing to varied learning requirements, environments, and preferences. These philosophies of education can be broadly classified into three categories.
All these philosophies of education share a commonality – they are based on a basic axiomatic premise which is typical of any of the established sciences – electric current only flows from a high voltage level to a lower one, demand goes down with an increase in price, two plus two always equals four. Analytics, on the other hand, is a different breed altogether. There is barely anything axiomatic about Analytics theory in that two plus two almost never equals four – you never know whether a Statistical model predicts well until you actually build it or you never know what delectable insights you might find in a root cause analysis which may be completely counter-intuitive to conventional expectation. If there is anything axiomatic in Analytics, it is utility. An analytical solution is not an end by itself but the means to an end – of more informed decision-making for businesses. And that has undeniable value. As George Box aptly put, “All models are wrong, but some are useful”.
Philosophy behind Analytics Training- Emergence that is analytics.
The pedagogy of Analytics calls for a hybrid philosophy that captures the best of all the philosophies. This includes an Essentialist flavour of in-depth instruction of fundamental tools and techniques, a dash of Perennialist viewpoint by showcasing case studies of what has worked in the real world, sprinkled with softer skills of analytical translation and espoused by the Behaviorist position. The mode of analytics training itself, is largely influenced by Progressivist and Constructivist philosophies; the student is instructed to develop avenues for self-learning on a continuous basis and expect every solution to arise from a process of patient discovery rather than deterministic means. Finally, the Reconstructionist climax of the philosophy ensures that there is an unwavering mindset to drive consumption aggressively, right from the design phase of the solutioning process. The sole purpose of analytics is to create real business value, failing to address which, will inevitably cause even the most advanced mathematical models integrated with machine learning or artificial intelligence capabilities, to find solace in someone’s Recycle Bin.
Riddled with idiosyncrasies of multiple internal and external agents, all businesses can be thought of as deceptively organized systems where Murphy’s Laws almost always hold good. Every new business decision may completely change the paradigm within which the decision was originally conceptualized. In other words, every solved problem may lead to an array of new problems to deal with. Therefore, our approach to analytics training accounts for the fact that data-driven decision-making may be a continuous pursuit. And to be well-trained to deal with such a situation, a data analyst needs to be well-versed with not just the tried-and-tested static solutions but also the dynamic process of how a never-before-seen ghost problem can be approached and solved through analytical means. It seems the sexiest job in the 21st Century comes with a disclaimer. Given this perspective, Analytics cannot be considered an exact science but a discipline that cyclically gives rise to bigger and better data-driven decision-making. Therefore, we have posited and adopted an Emergent philosophy of analytics training.
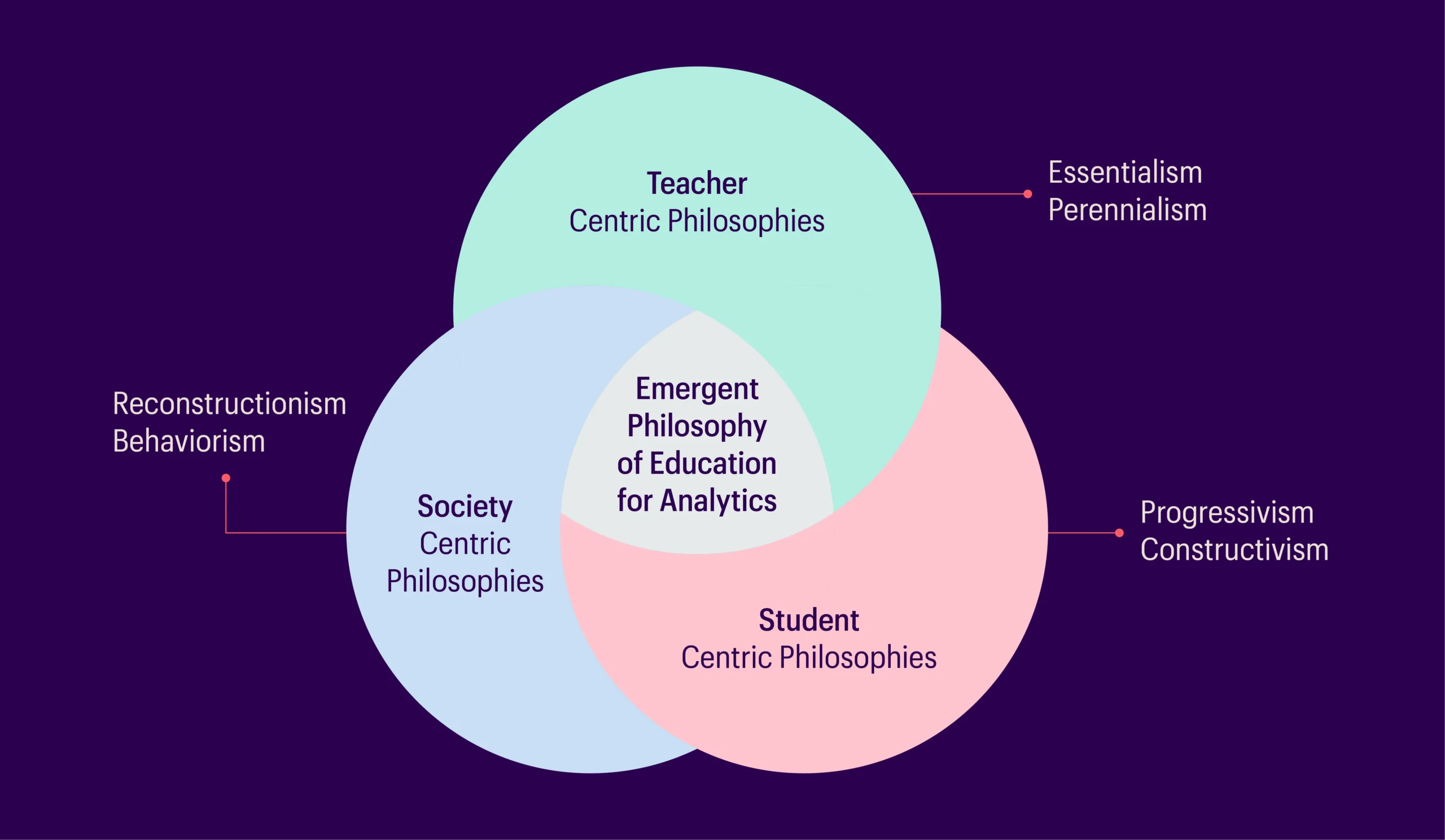
Key components of our Analytics Training offering:
At MathCo, learning how to make analytics succeed in an organization is core to our training offering. Here are key components that make our training exercises effective–
Problem packages:
The way one approaches estimation of a customer’s lifetime value in the retail domain is wildly different from how it is done in insurance; people will not switch insurers as often as they may switch their shampoo brand. The solution approach and consequent insights and recommendations are shaped by not only the domain but also the context that is unique to each business – particularly, the purpose that an analytics solution is supposed to serve.
Therefore, our Analytics Training offering materializes into what we call ‘problem packages’ that include not only in-depth coverage of analytical tools, techniques and technologies but also a primer on Problem Definition and Solution Design frameworks that we have perfected over years of experience across multiple domains. These frameworks are primarily designed to help analysts abstract and apply domain understanding to analytical solutioning, empathize with business stakeholders while keeping in mind the ultimate business purpose in order to come up with business-centric solutions.
Insights into Analytics best practices:
While there is much to learn about the wide array of analytical tools, techniques and technologies, data engineering platforms, methodologies and frameworks, the softer aspect of data analysis is also critical to deliver valuable decisions.
It would not be the case if business problems presented themselves in a clear, easy-to-understand template. An analyst will rarely find a ready-to-execute problem statement from the business and will have to uncover the right problem by asking the right questions i.e. find the problem behind the problem. Henry Ford seemed to have grasped this fact early on; he said, “If I really listened to what people were asking for, I would’ve ended up building faster horses”.
Probably the most important habit that a good analyst needs to inculcate is efficiency. Given the perspective above, it is obvious that not all solutions work – to do analytics one needs high motivation and the ability to deal with and learn from failed attempts. Every failed attempt sheds light on what the next approach should be. As one of the highly recommended analytics best practices, every now and again, one needs to eliminate hypotheses quickly and be able to start on a new one. And that requires a paradigm shift in a typical analyst’s mindset.
Therefore, Analytics Best Practices form a major portion of our training content which includes – the right way of asking questions, most efficient ways of doing research, how to best present analytical results, how to track multiple analysis iterations, and even the right way of brainstorming on new ideas.
Analytics Training philosophy- Storyboarding:
Storytelling is central to analytics solutions. It is critical to understand that it is not always easy to quantify the impact that analytics generates for a business. There is lot of notional value involved, as well as impact that may be seen sometime in the future. In addition, since analytics is the backbone of every function in an organization, its impact may be seen across different functions and in different ways – higher revenue, lower costs or shorter turnaround times etc. In such a scenario, it becomes imperative to not only build a good analytical solution but also to present it in a way that highlights the possible impact it will generate. Moreover, analytics seems like a black-box to most businesses and telling a good analytics story goes a long way in terms of creating a great stakeholder experience. A typical analytics story would include – the business problem at hand and how it was broken down to analytical problems, explored data sources and the accompanying observations, how the data was cleaned, transformed and prepared for analysis, the basis on which certain techniques were selected and why some of them did not work, techniques that worked and insights that can be gained, and finally, the suitable recommendations for the future.
Thus, an in-depth instruction using case studies and hands-on practice in incorporating storyboards into decks coupled with basic Powerpoint skills are core to our analytics training wherein participants are encouraged to showcase their creativity.
Validation through Consumption:
Nobody likes something that has no use. A lot of projects fall by the wayside due to unacceptance, leaving the analytics team dejected and low on morale. The inevitable attrition that follows leads to skill shortage and loss of context, over time. As a result, the credibility of analytics is lost in this vicious circle and analytics takes a hit. Thus, functions within an organization fall behind many years when it comes to analytics.
Driving Consumption Aggressively takes centre-stage when it comes to the way we deliver analytics training. We believe that every training module should generate something that can be used later or employed later. In other words, even a seemingly unidirectional activity like training should generate collateral benefit. A course on Statistics, for instance, will be futile unless participants are made to work with a real business problem, data and generate real insights that could serve as a proof-of-concept for our customers. A programming-heavy course on R or Python will make participants create a reusable code module that they can use in future live projects. Even an instruction in purely theoretical concepts will have a task of creating elementary training material on slightly more advanced concepts that can be used for later sessions. Essentially, the task or the assignment is thought of first. And then the course is designed around it.
Analytics Training philosophy – Analytics translators:
Analytics has its own language, which is very different from that spoken by businesses. It is not enough to be able to do analytics, but one must also understand what it takes to make analytics succeed in an organization. Therefore, the need for Analytics Translators.
Interpretation is a tricky business, especially with the advent of complex Machine and Deep Learning algorithms. After all, models are purely mathematical; a bunch of numbers fed into an equation and another bunch of numbers that come out of it. Therefore, a First Principles understanding of tools and techniques is essential and is enlisted as an analytics best practice if one must convince business stakeholders to take decisions based on those numbers. Moreover, empathizing with stakeholders is equally important – for example, in a performance driver analysis, there is no point in identifying those drivers, no matter how mathematically significant, if they cannot be controlled by the business.
All advanced models tend to give up at “there isn’t enough data available to generate accurate forecasts”. That is where human creativity steps in. Machine Learning models are notorious for generating sub-zero sales forecasts for severely fluctuating data, even with the most advanced techniques. It takes human intervention to design a solution, so it does not appear as if the company is paying customers to buy its products. The truth is, there may never be enough data, but an analyst should be able to eyeball analytical outputs and make some sense out of those numbers.
In addition, for a translator, it is critical to understand the purpose of analytics itself i.e. Analytics is a means to an end. The goal is not to get the most accurate analytical model that can create the greatest business impact with the given resources, but the reverse – what will it take to create the greatest business impact? The ability to answer that question involves thinking holistically about the business and understanding that there is no single way to solve a business problem.
Our success stories:
Our emergent philosophy has brought us success with a multitude of customers. Some of the most interesting ones include –
- We brought about an org-wide sensitization of analytics and how it can help with decision-making for one of the world’s largest CPG companies. The analytics training covered over 60,000 employees spread across multiple geographies and business functions through classroom sessions, webinars and self-learn courses.
- We identified specific needs for Advanced Analytics in the ecosystem of one of the world’s largest retailers and conducted classroom sessions for 200+ existing employees as well as their incoming batch of fresh graduates.
- Responding to end users’ evolving demand for off the shelf and plug and play products that can be customized to business challenges, TheMathCompany has been conducting expert-led extensive training classes on contextual AI. Clients’ expectations to exercise absolute control on the solutions that they procure is paving the way for the advent and popularity of contextual AI.
- We assisted with demand generation and evangelization across multiple geographies through roadshows, case study showcases and conferences, for the world’s largest beverage manufacturer.
- We have been conducting a specialized analytics training program – Experiential Learning – for one of the largest Commercial Insurers in South-East Asia, for over 2 years. The training program is a combination of classroom instruction and execution assistance on live analytics projects. A proof-of-concept that resulted from our classroom course is a collateral benefit that turned into a live project and continues to generate revenue for the business.
Conclusion:
Today, forward-thinking businesses are cued in on the need to proactively train their teams so they can tackle specific and sticky data challenges. It’s essential to boost data literacy alongside, to allow analytics to permeate across all levels in the organization and foster a data-driven culture that collectively impacts business outcomes.